Driving a Culture of Data Science
The first principle is that you must not fool yourself and you are the easiest person to fool. - Richard Feynman
Data Science is having its 'day' in the sun. This strange stew of computer science, algorithmic skills, hacking abilities, statistical inferencing, probabilistic modeling and scientific enquiry which started off as a rebranding of statistical work became a bona-fide job title only in 2008. Yet, it is now the 'sexiest job of the 21st century'. Amidst all the hoopla there is a lot of confusion on what constitutes a data scientist. However tempting it may be to try and define a high-priesthood of data science, the fuzziness comes from the fact that different businesses require and therefore rightly organize themselves around different flavors of data science. However we choose to define it, data science requires a fundamentally interdisciplinary mindset to problem solving. Data science at MSD is centered around building data products using machine learning, statistical inferencing and modeling of domain knowledge. Some musings on our data science culture -
The soapbox comes before the speech
A reliable data platform is the cornerstone of an effective data science team. Our data platform instruments every product with usage data, pipelines and consolidates them strategically at different fidelities for use in data science workflows. Careful deliberation about scale is a key player in our data architecture. Data comes in different shapes and sizes and the platform must open doors for them all.
How I Learned to Stop Worrying and Love the Error
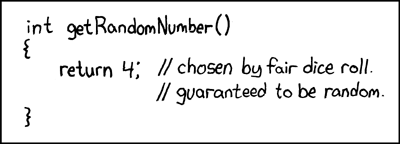
Building a data-driven culture early on and embedding this deep into the DNA of the company is important for effective machine learning and data science. Data Science projects are characterized by weak contracts and experimental iterations defined by measures of accuracy and variability and various tradeoffs. A process of scientific inquiry and decision-making must be followed to allow the algorithms to evolve.
All models are wrong, but some are useful
"Now it would be very remarkable if any system existing in the real world could be exactly represented by any simple model. However, cunningly chosen parsimonious models often do provide remarkably useful approximations. For example, the law PV = RT relating pressure P, volume V and temperature T of an "ideal" gas via a constant R is not exactly true for any real gas, but it frequently provides a useful approximation and furthermore its structure is informative since it springs from a physical view of the behavior of gas molecules.
For such a model there is no need to ask the question "Is the model true?". If "truth" is to be the "whole truth" the answer must be "No". The only question of interest is "Is the model illuminating and useful?". -[Box, 1978]
Decades later this is still relevant to data science today. We begin by building an empirical model which makes generalizations about the environment it operates upon based on evidence and prior knowledge, then revises these beliefs under the right conditions.
Model knowledge not information
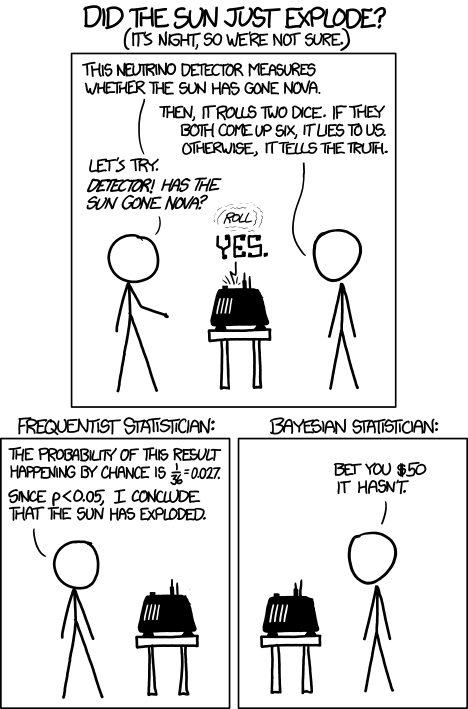
In order to extract true signals from a firehose of various modalities of data including visual, textual, behavioral etc. coupled with human expertise we must understand the underlying semantics of entities and their interconnections. Building abstractions of knowledge and connecting the causal dots is key to mining the patterns that will inform our data products.
Learning on the job
Insanity: doing the same thing over and over again and expecting different results. - Einstein
Unless you've been living under a rock lately you would have heard of Deep Learning - the Jack Bauer of AI, playing a key role in many of the recent technical milestones such as the AlphaGo win. Often missing from popular commentary, the role of reinforcement learning, for instance Monte Carlo Tree Search in AlphaGo. Building intelligent agents that rationalize and course-correct on the fly is a key piece of the AI puzzle.
Avoiding filter bubbles with 'Informed Serendipity'
A personalized 'nudge' is the de-facto icing on all our data products. Personalization, when done right, serves as a veritable force multiplier, especially in e-commerce where multiple websites are vying for customers' limited attention. However it can quickly devolve into a caricature without a deeper conceptual understanding of customer needs. Enabling delightfully surprising yet relevant discovery is a key objective of our algorithms.
Lessons on not picking up nickels in front of a steamroller
A predictive modeling mindset is necessary to detect the seasonality and trends intrinsic to the data which in turn paves the way for proactive rather than reactive data products. Running blind without sensible forecasting for foreseeable spikes and expected seasonal behavior leads to enormous missed opportunities for conversion. For instance - running controlled experiments during festive seasons resulting in significant loss of revenue.
Seeing the forest through the trees
Ideas come and go, stories stay - Nassim Nicholas Taleb
In order to transform data into product behavior we must craft a compelling story to explain the data in a meaningful way. Data storytelling is so crucial that it is often said to be the Data Scientists' 'real job' - a gross oversimplification but nonetheless important. Transcending counting and finding the compelling narrative that lies beneath is a major part of our data products' life-cycle.
Can I get the icon in cornflower blue?
'In God we trust; all others bring data. - W. Edwards Deming
Sometimes seemingly innocuous things such as the color of a button can have a more significant impact than hyper-tuning for algorithmic efficiency in a vacuum. As a team we tend to have a healthy dose of skepticism towards decision-making that evades quantitative or qualitative assessment. Running controlled experiments to understand what makes users tick is tricky but essential to the evolution of the algorithm. Every heuristic that affects the behavior of our algorithms is regularly backed up and fine-tuned with data points.
These are some of our thoughts in the 'path to less wrongness'. A year and a half in the trenches - this is still early days for our data science team. As our data evolves so will our beliefs and sweeping generalizations. Watch this space for more.